Name: Predicting Individual Differences in Response to Sleep Loss: Application of Current Techniques.
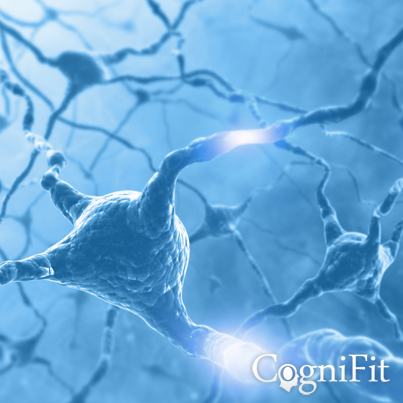
Predicting Individual Differences in Response to Sleep Loss: Application of Current Techniques
Scientific publication on cognitive evaluation of pilot fatigue
Conveniently manage research patients from the researcher's platform
Evaluate and train up to 23 cognitive skills for your study participants
Check and compare participants' cognitive development for your study data
Authors: Joseph F. Chandler1, Richard D. Arnold1, Jeffrey B. Phillips1, Ashley E. Turnmire1.
- 1. Naval Medical Research Unit.
Journal: Aviation, Space and Environmental Medicine (2013), vol. 84 (9): 927-937.
References to this article (APA style)::
- Chandler, J. F., Arnold, R. D., Phillips, J. B., Turnmire, A. E. (2013). Predicting Individual Differences in Response to Sleep Loss: Application of Current Techniques. Aviat Space Environ Med., vol.84, pp.927-937.
Study Conclusion
CogniFit can measure highly relevant variables to predict individual user fatigue performance. This can help reduce the risk of accidents for military pilots and civilians, as tiredness is a recurring variable in various types of accidents. . Including some variables, such as response time (p=0.009), short-term memory (p=0.023), divided attention (p=0.026) or cognitive flexibility (p=0.002) in predictive models, the percentage of variance explained goes from 13.8% to 35.7%.
Study Summary
The fatigue is one of the main factors that puts safety at risk during military transport. Models predicting the response to fatigue have been made, but are not yet sufficiently accurate, as they do not take into account the individual differences in fatigue sensitivity. Instead, it is proposed that the predictive capacity of these models could be improved if cognitive measurements were performed using tools such as CogniFit and oculometric to account for individual differences.
The different cognitive and oculometric variables were measured in rested participants and every 3 hours during a 25-hour wakefulness process (so the group and individual scores were obtained). It was also possible to compare actual performance with expected performance. The results indicated that by adding these measures to the pre-existing models, they went from explaining 13.8% to 35.7% of the variance. This means that, using CogniFit and other measures to detect individual differences, can greatly improve the prediction of performance during fatigue and thus improve safety.
Context
Fatigue due to lack of sleep is one of the main risks they face in both military and civilian transport. In principle, the solution to these problems would be the sleeping properly and/or resort to drugs. However, sometimes this is not enough. Much of this can be prevented by predicting a person's performance or directly measuring an individual's ability to act at the right time. This prediction model, on the other hand, has a moderate effectiveness by itself.
The relative lack of success of this model may be because it assumes that all individuals have a similar circadian rhythm and response to fatigue, while studies suggest that individual differences in these settings are significant. Some of the aspects that interfere with this response to fatigue are a person's cognitive functioning.
Therefore, an increase in the effectiveness of the predictive model could be expected if we include measures that take into account individual differences, such as cognitive and oculometric measures.
Methodology
Participants
The participants consisted of 15 volunteers from the Naval Aviation Preflight Indoctrination (API) program on board the Naval Air Station Pensacola, serving military personnel (13 men and 2 women, with an average age of 24.7 and 21.5 years respectively). To participate in the study, alcohol, caffeine, and tobacco use were controlled, and they had to be free of neurological, psychiatric, or sleep-related problems.
Procedure
A design of repeated measures was applied to learn about the effects of sleep deprivation on cognitive and oculometric performance, both at a group and individual levels. First, the baseline was recorded and then the data was taken during sleep deprivation.
Statistical Analysis
The analysis was carried out in three steps:
- Step 1: A series of ANOVAs were performed for each criterion and predictor variable measured in each trial. This determined what variables showed changes over time.
- Step 2: A series of bivariate linear hierarchical models with fixed and random effects were carried out with the objective of predicting when fatigue would produce a lower yield and, in turn, discovering undetected differences in the group analysis level. A group effect (p<0.05) and individual differences within that overall effect (0<0.05) were detected. Following this, a multivariate multi-hierarchical linear model was carried out to know which predictor variables shared explanatory variance at the statistical level and relationship at the conceptual.
- Step 3: A series of general linear models were made from the significant predictor variables of the previous step. Thus, the aim was to know the predictive capacity of the model by taking into account cognitive and oculometric factors.
Results and Conclusions
In Step 1 of the data analysis, the effects of group were obtained. It was observed that there were significant effects on response time (p=0.009), short-term memory (p=0.023), divided attention (p=0.026) and cognitive flexibility (p=0.002). With fatigue, there was a reduction in the performance of these cognitive abilities, so they were taken into account as predictive variables in the next step. In Step 2 of the analysis, the individual differences were obtained through significant relationships between different variables with fixed or random effects. Step 3 of data analysis, it was observed that when only classical prediction measures were used, predictions could only account for 13.8% of the variance. By contrast, adding significant cognitive variables, predictions could account for 35.7% of the variance.
These results indicate that adding some fatigue-sensitive variables to the usual predictive models, such as CogniFit measures, can help us predict more accurately when performance will be affected by fatigue. Knowing this information can be very useful to prevent accidents and take precautionary measures in both military and civilian airplanes.